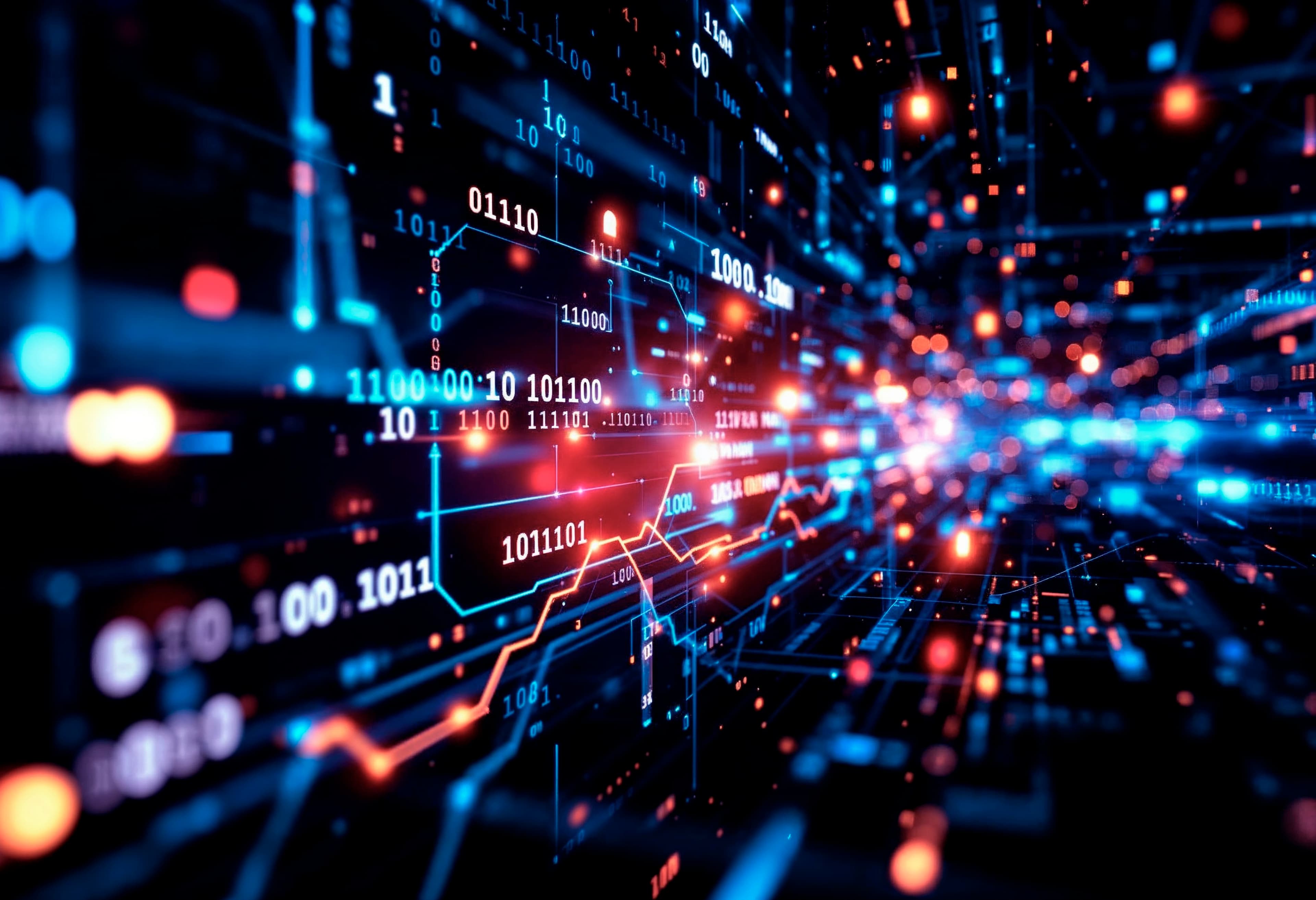
volatility
The Key to Navigating Financial Crises: Adjusting for Volatility
April 4, 2025 – Palmarium AI
In recent weeks, market volatility has surged, with the VIX, the primary volatility indicator, climbing above 40 points today. This reflects heightened uncertainty about the global economy’s future, as top-tier banks have raised the probability of a recession to up to 60%. While slow economic growth and market disruptions from new U.S. tariff policies — and the global response — may be contributing factors, let us set aside the causes and focus on how to navigate market crises with an active portfolio management mindset. The approach we’ll take is the volatility-managed portfolio one, following the paper Volatility managed portfolios by Moreira and Muir [1]. Here, the authors present a systematic approach to enhancing risk-adjusted returns by dynamically adjusting portfolio exposure based on volatility. The core insight is that scaling factor investments inversely to their recent volatility — reducing exposure when volatility is high and increasing it when volatility is low — improves Sharpe ratios. Its implications challenge traditional asset pricing models by showing that volatility timing generates significant alpha despite the absence of proportional risk premium adjustments during volatile markets. The paper provides a framework for designing dynamic strategies that adapt to volatility regimes. Its simplicity and robustness by using lagged realized variance makes it applicable to factor investing, risk parity, and hedging. For quants, this work highlights the value of conditional risk management — even in efficient markets — and offers a tractable tool for enhancing factor-based strategies. While the paper focuses on alpha generation — and our internal research confirms its feasibility — we have extended the framework to prioritize Sharpe ratio enhancement through risk mitigation rather than return maximization. This is achieved by integrating volatility into a market stress indicator, which activates a reduction in market exposure when triggered. The core objective is volatility reduction, accomplished by dynamically adjusting the long-short ratio while maintaining a fixed total allocation (TA = L + S, where L and S represent absolute long and short allocations). Our analysis reveals that long-short strategies exhibit an optimal allocation point — not necesarily market neutrality — where time-averaged volatility is minimized. To identify this point, we leverage in-sample backtest results to calibrate the ratio. As illustrated in the figure below, the resulting allocation typically balances risk efficiency with exposure constraints. The figure displays an ilustration of the explicit volatility (standard deviation of the daily returns) as a function of the Long short ratio L/S, with L and S, the long and short allocations in absolute value. The total allocation TA (TA = L + S) is fixed, so that the volatility is not modified by the amount invested. There is a sweet spot in the graph where the volatility is at the lowest. When the volatility signal is triggered, the strategy transitions from its standard L/S allocation to an optimal risk-adjusted position. The concept is straightforward yet powerful: during periods of heightened market uncertainty, we systematically reduce exposure to mitigate portfolio volatility. Out-of-sample backtests demonstrate that this approach enhances the Sharpe ratio over intermediate time horizons. For example, the signal was triggered several times during the 2022 out-of-sample results, boosting the backtest annual returns by more than 20%. Furthermore, integrating the volatility signal with our proprietary volatility forecasting model amplifies these improvements [2]. The past week’s events Our propietary volatility signal operates as a binary indicator — either “on” or “off” — triggered by specific market conditions. When activated, it prompts a rebalancing of the portfolio to optimize the allocation between long and short positions, aiming to minimize overall volatility. By systematically adapting to market conditions, our strategy minimizes drawdowns during crises while capitalizing on periods of stability to deliver superior risk-adjusted returns. Our volatility signal activated on January 22 2025 and has been “on” since then. Year-to-date, our flagship strategy has delivered a 1% return versus the SPY’s decline of over 13%, underscoring its resilience in turbulent conditions. References: [1] Moreira, A. and Muir, T. (2017), Volatility-Managed Portfolios. The Journal of Finance, 72: 1611–1644. https://doi.org/10.1111/jofi.12513 [2] Machine Learning & Volatility Forecasting: Avoiding the Look-Ahead Trap
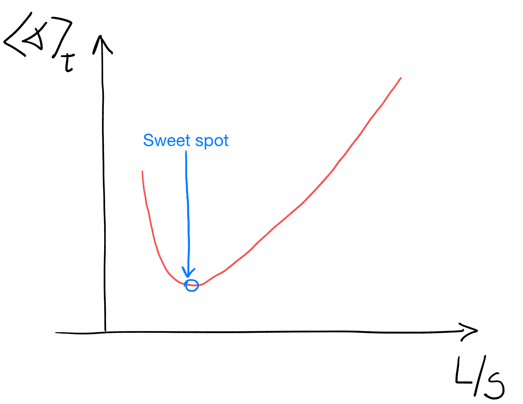
April 4, 2025 – Palmarium AI
In recent weeks, market volatility has surged, with the VIX, the primary volatility indicator, climbing above 40 points today. This reflects heightened uncertainty about the global economy’s future, as top-tier banks have raised the probability of a recession to up to 60%. While slow economic growth and market disruptions from new U.S. tariff policies — and the global response — may be contributing factors, let us set aside the causes and focus on how to navigate market crises with an active portfolio management mindset. The approach we’ll take is the volatility-managed portfolio one, following the paper Volatility managed portfolios by Moreira and Muir [1]. Here, the authors present a systematic approach to enhancing risk-adjusted returns by dynamically adjusting portfolio exposure based on volatility. The core insight is that scaling factor investments inversely to their recent volatility — reducing exposure when volatility is high and increasing it when volatility is low — improves Sharpe ratios. Its implications challenge traditional asset pricing models by showing that volatility timing generates significant alpha despite the absence of proportional risk premium adjustments during volatile markets. The paper provides a framework for designing dynamic strategies that adapt to volatility regimes. Its simplicity and robustness by using lagged realized variance makes it applicable to factor investing, risk parity, and hedging. For quants, this work highlights the value of conditional risk management — even in efficient markets — and offers a tractable tool for enhancing factor-based strategies. While the paper focuses on alpha generation — and our internal research confirms its feasibility — we have extended the framework to prioritize Sharpe ratio enhancement through risk mitigation rather than return maximization. This is achieved by integrating volatility into a market stress indicator, which activates a reduction in market exposure when triggered. The core objective is volatility reduction, accomplished by dynamically adjusting the long-short ratio while maintaining a fixed total allocation (TA = L + S, where L and S represent absolute long and short allocations). Our analysis reveals that long-short strategies exhibit an optimal allocation point — not necesarily market neutrality — where time-averaged volatility is minimized. To identify this point, we leverage in-sample backtest results to calibrate the ratio. As illustrated in the figure below, the resulting allocation typically balances risk efficiency with exposure constraints. The figure displays an ilustration of the explicit volatility (standard deviation of the daily returns) as a function of the Long short ratio L/S, with L and S, the long and short allocations in absolute value. The total allocation TA (TA = L + S) is fixed, so that the volatility is not modified by the amount invested. There is a sweet spot in the graph where the volatility is at the lowest. When the volatility signal is triggered, the strategy transitions from its standard L/S allocation to an optimal risk-adjusted position. The concept is straightforward yet powerful: during periods of heightened market uncertainty, we systematically reduce exposure to mitigate portfolio volatility. Out-of-sample backtests demonstrate that this approach enhances the Sharpe ratio over intermediate time horizons. For example, the signal was triggered several times during the 2022 out-of-sample results, boosting the backtest annual returns by more than 20%. Furthermore, integrating the volatility signal with our proprietary volatility forecasting model amplifies these improvements [2]. The past week’s events Our propietary volatility signal operates as a binary indicator — either “on” or “off” — triggered by specific market conditions. When activated, it prompts a rebalancing of the portfolio to optimize the allocation between long and short positions, aiming to minimize overall volatility. By systematically adapting to market conditions, our strategy minimizes drawdowns during crises while capitalizing on periods of stability to deliver superior risk-adjusted returns. Our volatility signal activated on January 22 2025 and has been “on” since then. Year-to-date, our flagship strategy has delivered a 1% return versus the SPY’s decline of over 13%, underscoring its resilience in turbulent conditions. References: [1] Moreira, A. and Muir, T. (2017), Volatility-Managed Portfolios. The Journal of Finance, 72: 1611–1644. https://doi.org/10.1111/jofi.12513 [2] Machine Learning & Volatility Forecasting: Avoiding the Look-Ahead Trap
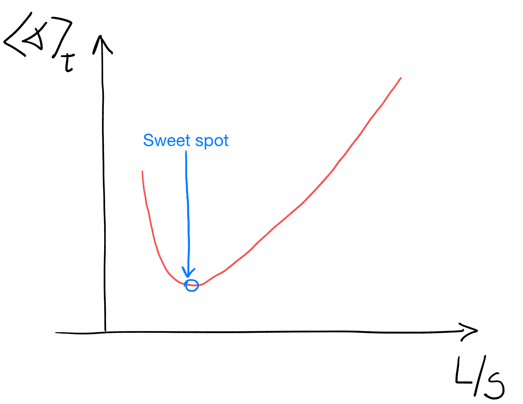
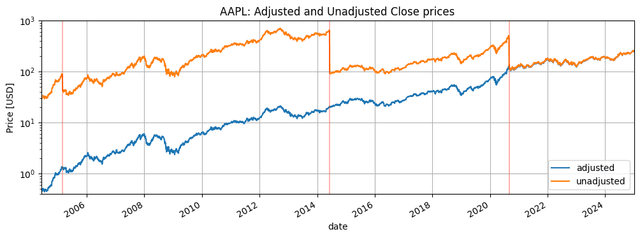
March 27, 2025 – Palmarium AI
A well-conducted backtest should accurately reflect how a trading strategy would have performed between two points in time, using only the information available at the start of the period. This approach ensures that the backtest is free from look-ahead bias, a common pitfall that can skew results by incorporating future data [1]. One critical aspect of avoiding this bias is the proper use of adjusted and unadjusted price data series. The term “adjusted” refers to the process of modifying an asset’s price retroactively to account for events like dividends and stock splits. This adjustment involves subtracting dividends or applying a split factor to maintain the market value of a position as if it had been held continuously. Unadjusted data, on the other hand, reflects the raw price that it was seen at a given moment [1]. The following figure displays an example of these time series for Apple Inc. Adjusted (blue) and Unadjusted (orange) close prices for APPLE INC (ticker: AAPL). The vertical red lines correspond to AAPL splits events. While the unadjusted line reflects the actual close value seen at that particular date, the adjusted line is smooth during the splits events, which is useful for a quick return computation. Both values are useful when backtesting, but some considerations must be taken, so here is a practical guide on when to use adjusted and raw price data in a backtest. Historical return series Returns on a given asset can be computed using either adjusted or unadjusted prices. While intraday returns (i.e., returns within a trading day, such as hourly returns) can be computed straightforwardly with both values as where P denotes the price, t (subindex) represents the end time of the period, and ΔT (subindex) represents the time interval, such that t−ΔT marks the start of the period and t marks the end; daily or higher-period returns can only be computed using adjusted prices with this formula. To obtain these returns with unadjusted price series, split and dividend corrections must be taken into account [1]. Shares’ Quantity The stock’s shares quantity is crutial to accurately describe the state of a portfolio at a given time with metrics such as AUM, P&L and several risk measurements. In a backtest it is typical to construct the portfolio’s share quantities from asset weights as where the subindex i indicates the company, ω is a fractional weight (how much of the portfolio is composed by this particular asset), and Eq is the total equity held at time t. Here P can either be an adjusted or unadjusted price, and as a result Q can be either an adjusted or unadjusted share quantity. If the value inside the int function is (in absolute value) less than one, then Q is set to 0, and the portfolio wouldn’t hold any share on this asset. Consider an hypothetical portfolio with an AUM of USD 1M (Eq = 1e6), were the weight for a particular asset X is 1% (ω = 0.01). This porfolio should hold approximately USD 10000 worth of X (ω.Eq = 1e4). If X is AAPL, then the adjusted Q in early 2008 is higher than 1000 (as the adjusted price is lower than USD 10), while the unadjusted Q is less than 100 (as the unadjusted price is higher than USD 100). The AAPL position in USD remains unchanged, but the share quantity differs significantly. Now consider the same example with TOP SHIPS Inc (TOPS), for which the time series are shown in the figure below. While the unadjusted quantity for early 2008 would be around 10000, the adjusted one would be 0. So if we compute the adjusted shares’ quantity, the portfolio would have no positions on TOPS. Adjusted (blue) and Unadjusted (orange) close prices for TOP SHIPS Inc (ticker: TOPS). The vertical red lines correspond to TOPS reverse splits events. TOPS has undergo several reverse splits, and the adjsuted and unadjusted price time series are several orders of magnitude appart. Trading costs There are several trading costs, including trading commissions, bid-ask spreads, and slippage [1]. In this section, we will focus on trading commissions following the two examples set in the previous section. Different brokers charge different commission schemes. However, it is common for trading commissions to be calculated based on share quantity. For example, Interactive Brokers Pro commissions range from USD 0.0005 to USD 0.0035 per share [2]. When trading commission costs depend on share quantity, this amount should be computed using unadjusted time series since this reflects the real quantity bought or sold at any given time. Cross-sectional comparissons and stock filtering Cross-sectional comparissons (comparing across a stock universe on a given fixed time) is a common practice in trading strategies [3]. Here, the correct price series is the raw, unadjusted one. This is quite simple to understand with an example. Let us compare two stocks, AAPL and TOPS at January 2010. If the comparison is done with adjusted data then TOPS’s close price is greater than AAPL’s close price. But the opposite is true for the unadjusted price. At January 2010 what we would see is the raw, unadjusted price, and so AAPL’s close price would be greater than TOPS. This can be generalize to cross-sectional analysis. Conclusions When deciding whether to use adjusted or unadjusted data in backtests, consider these points: Cross-sectional comparisons require unadjusted data. Time-series analysis concerning a single asset can use either adjusted or unadjusted data but tends to be more straightforward with adjusted data. Trading costs dependent on share quantity must be computed using raw prices since adjustments affect share quantities due to splits and dividends. References [1] Isichenko, Michael. Quantitative Portfolio Management: The Art and Science of Statistical Arbitrage. Hoboken, NJ: Wiley, 2021. [2] https://www.interactivebrokers.com/en/trading/products-stocks.php [3] Zarattini, Carlo, Alberto Pagani, and Cole Wilcox. Does Trend-Following Still Work on Stocks?. Available at SSRN, 2025. Palmarium backtest policies At Palmarium AI, we adhere to strict policies concerning our backtesting practices. We submit our backtests to a thorough peer-review internal process and compare results with independently developed backtesting code. This enhances reliability and integrity in our risk assessments and strategy evaluations.
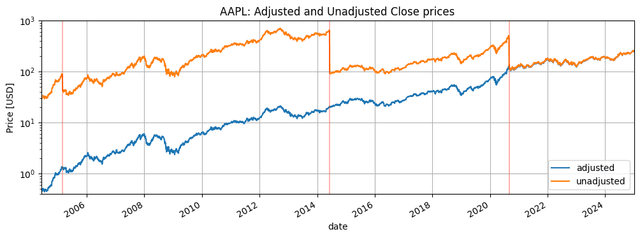
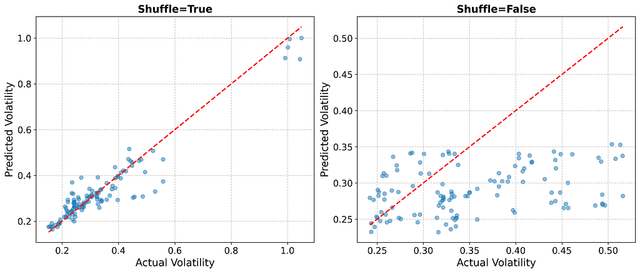
February 28, 2025 – Palmarium AI
Volatility forecasting is a pillar of risk management, helping investors and financial institutions assess market uncertainty and make informed decisions. Over time, various models have been developed to predict volatility, ranging from traditional econometric approaches like GARCH [1] to the increasingly popular machine learning (ML) techniques [2]. While ML models offer powerful pattern recognition and adaptability, their application in finance requires careful consideration to avoid common pitfalls like look-ahead bias. This is exactly what occurs in the model presented on this Medium article. There, a Python implementation of one-month-ahead volatility forecasting model using Random Forest is presented. Based on the results shown, you might think that the model accurately predicts next month’s volatility — after all, that’s the claim, and the data may seem aligned with the results. However, there is a significant look-ahead bias in their code, which compromises the validity of the forecast. Look-ahead bias occurs when a model or strategy inadvertently uses information that would not have been available at the time of making a decision. To set an example, imagine you’re a time-traveling stock trader who goes back to February 2020, knowing about the upcoming COVID-19 pandemic and March market crash. You use this future knowledge to short the market heavily, resulting in millions in profits when March arrives. This scenario illustrates look-ahead bias: making decisions using information that wasn’t actually available at the time, leading to unrealistically positive results (in your backtest) that wouldn’t be achievable in real-world trading. In this context, we’ll explore how easy it is to unintentionally introduce future information into the training process. Let’s dive into the code. 1. Data Collection and Preparation Here, the price data is retrieved from yfinance, and thepandas.DataFrameto be used in the Random Forest model is set up. import yfinance as yf import pandas as pd import numpy as np import matplotlib.pyplot as plt from ta.momentum import RSIIndicator from ta.trend import MACD # Download historical stock data ticker = 'AAPL' # Apple Inc. data = yf.download(ticker, start="2020-01-01", end="2023-01-01", auto_adjust=False) data['Returns'] = data['Adj Close'].pct_change() # Daily returns # Calculate historical volatility window = 21 # 21 trading days data['Volatility'] = data['Returns'].rolling(window=window).std() * np.sqrt(252) # Annualized volatility # Feature: 21-day rolling mean (Moving Average) data['MA_21'] = data['Adj Close'].rolling(window=21).mean() # Feature: RSI rsi = RSIIndicator(data['Adj Close'].squeeze(), window=14).rsi() data['RSI'] = rsi # Feature: MACD macd = MACD(data['Adj Close'].squeeze()) data['MACD'] = macd.macd_diff() # Drop NaN values data.dropna(inplace=True) data.columns = data.columns.get_level_values(0) Explanation: The features used include daily returns, historical volatility, moving averages of stock prices, and two technical indicators: Relative Strength Index (RSI) and Moving Average Convergence Divergence (MACD). 2. Building and training the Machine Learning Model A Random Forest Regressor is used to forecast future volatility, with the target variable defined as the volatility one month ahead (volatility shifted by -21). The look-ahead bias in this model is introduced during the data split for training and testing using the train_test_split function from scikit-learn. Specifically, the default setting for the shuffle parameter (shuffle=True) is not changed, which results in random shuffling of the dataset before splitting. This inadvertently allows future information to leak into the training data, leading to a biased model. Let’s examine how the model’s results change by simply adjusting the value of this parameter. import numpy as np import matplotlib.pyplot as plt from sklearn.model_selection import train_test_split from sklearn.ensemble import RandomForestRegressor from sklearn.metrics import mean_squared_error # Create target variable data['Future_Volatility'] = data['Volatility'].shift(-21) # Forecast 21 days ahead # Drop NaN values data.dropna(inplace=True) # Features and target X = data[['MA_21', 'RSI', 'MACD', 'Volatility']] y = data['Future_Volatility'] # Function to train model and predict values def train_and_predict(shuffle_value): """Train the model and return actual and predicted values.""" # Split data with specified shuffle parameter X_train, X_test, y_train, y_test = train_test_split(X, y, test_size=0.2, random_state=42, shuffle=shuffle_value) # Train Random Forest model model = RandomForestRegressor(n_estimators=100, random_state=42) model.fit(X_train, y_train) # Predict on test data y_pred = model.predict(X_test) return y_test, y_pred # Function to evaluate model performance def evaluate_model(y_test, y_pred): """Calculate and return RMSE of the model.""" rmse = np.sqrt(mean_squared_error(y_test, y_pred)) return rmse # Train and evaluate for shuffle=True y_test_shuffled, y_pred_shuffled = train_and_predict(shuffle_value=True) rmse_shuffled = evaluate_model(y_test_shuffled, y_pred_shuffled) print(f"RMSE (Shuffle=True): {rmse_shuffled}") # Train and evaluate for shuffle=False y_test_ordered, y_pred_ordered = train_and_predict(shuffle_value=False) rmse_ordered = evaluate_model(y_test_ordered, y_pred_ordered) print(f"RMSE (Shuffle=False): {rmse_ordered}") Explanation: Here we train the RF model with the two shuffle values: shuffle=Trueand shuffle=False. 3. Evaluating the Machine Learning Model # Function to plot actual vs predicted values def plot_actual_vs_predicted(y_test, y_pred, shuffle_value, ax): """Plot the actual vs predicted values as a scatter plot.""" # Plot actual vs. predicted values ax.scatter(y_test, y_pred, alpha=0.5) # Plot the ideal line (45-degree line) ax.plot([min(y_test), max(y_test)], [min(y_test), max(y_test)], color='red', linestyle='--', linewidth=2) # Ideal line # Set titles and labels ax.set_title(f"Shuffle={shuffle_value}", fontsize=16, fontweight='bold') ax.set_xlabel("Actual Volatility", fontsize=14) ax.set_ylabel("Predicted Volatility", fontsize=14) # Improve ticks and grid ax.tick_params(axis='both', which='major', labelsize=12) ax.grid(True, linestyle='--', alpha=0.7) # Create subplots fig, axes = plt.subplots(1, 2, figsize=(14, 6)) # Two subplots in one row # Plot for shuffle_value=True plot_actual_vs_predicted(y_test_shuffled, y_pred_shuffled, shuffle_value=True, ax=axes[0]) # Plot for shuffle_value=False plot_actual_vs_predicted(y_test_ordered, y_pred_ordered, shuffle_value=False, ax=axes[1]) # Adjust layout for clarity plt.tight_layout() plt.show() Impact of Data Shuffling on Volatility Forecasting: predicted vs. actual volatility when using Random Forest with shuffle=True (left) and shuffle=False (right). The red dashed line represents an ideal prediction. Notice how shuffling introduces look-ahead bias, leading to overly optimistic results. The figure above highlights the impact of data shuffling on volatility forecasting with Random Forest. When shuffle=True (left plot), the predicted values appear closely aligned with actual volatility, suggesting strong model performance. However, this is misleading due to look-ahead bias. In contrast, when shuffle=False (right plot), the predictions deviate significantly from actual values, reflecting a more realistic out-of-sample performance. This is further quantified by the 70% increase in Mean Squared Error (MSE) in the test set (on average), demonstrating how improper data handling can severely distort forecasting accuracy. Another way of looking at the difference is plotting the target and predicted time series, like it was done in the original post. # Function to plot time-series def plot_series(y_test, y_pred, title, ax): """Plot actual vs predicted volatility in a given subplot.""" ax.plot(y_test.values, label='Actual Volatility', color='blue') ax.plot(y_pred, label='Predicted Volatility', color='red') ax.set_title(title, fontsize=16, fontweight='bold') ax.legend() ax.grid(True, linestyle='--', alpha=0.7) ax.set_xlabel("Time", fontsize=14) ax.set_ylabel("Volatility", fontsize=14) ax.tick_params(axis='both', labelsize=12) # Create figure with two subplots fig, axes = plt.subplots(1, 2, figsize=(14, 6)) # Plot for shuffle=True plot_series(y_test_shuffled, y_pred_shuffled, "Shuffle=True (Biased)", axes[0]) # Plot for shuffle=False plot_series(y_test_ordered, y_pred_ordered, "Shuffle=False (Realistic)", axes[1]) # Adjust layout plt.tight_layout() plt.show() Comparison of Actual vs Predicted Volatility: The left plot shows the model performance with shuffle=True, leading to a look-ahead bias. The right plot shows the results with shuffle=False, reflecting a more realistic, unbiased forecasting approach. As seen in the figure, there is a significant difference between introducing a look-ahead bias (left) and simulating a real-life scenario (right). The plot on the left shows predictions vs. target volatility values from the test set, where the data comes from a shuffled dataset (shuffle=True). Here, the x-axis doesn’t represent time but rather an arbitrary index. On the right, with shuffle=False, the model respects the natural order of time, and while the predictions are less accurate, the x-axis accurately reflects time, which mirrors real-world forecasting conditions. 4. Final Remarks Setting shuffle=True in time series forecasting introduces look-ahead bias because it randomly shuffles the dataset before splitting it into training and test sets. This means that future data points can end up in the training set while earlier data points are in the test set. Since time series data has a natural temporal order (where past values influence future values), shuffling disrupts this order and allows the model to learn patterns that include information from the future — something that would be impossible in real-world forecasting. As a result, the model appears to perform much better than it actually would in a true out-of-sample scenario, leading to overly optimistic results that won’t hold in live trading or real applications. Forecasting volatility of financial assets is undoubtedly a challenging task, particularly when predicting over longer time horizons [3]. The inherent unpredictability of markets, coupled with the complexity of capturing the right features, makes it difficult to achieve consistent accuracy. Moreover, when applying machine learning models to volatility forecasting, it’s crucial to be vigilant about the potential leakage of future data during the training process. Even small slips, such as unintentional shuffling of data, can lead to inflated performance metrics and undermine the reliability of the model in real-world scenarios. Disclaimer: here we use yfinance for easy access to financial data, but we do not recommend using yfinance datasets for backtesting, as it displays other types of look-ahead bias. References: [1] Engle, R. F. Autoregressive Conditional Heteroskedasticity with Estimates of the Variance of United Kingdom Inflation. Econometrica, 50(4), 987–1007 (1982). [2] Zhang, L., Xie, L., & Zheng, Y. Forecasting financial volatility with deep learning. Journal of Computational Finance, 23(2), 1–22 (2019). [3] Andersen, T. G., & Bollerslev, T. Deutsche mark–dollar volatility: Intraday activity patterns, macroeconomic announcements, and longer run dependencies. Journal of Finance, 53(1), 219–265 (1998). Written by Palmarium AI’s Quant team
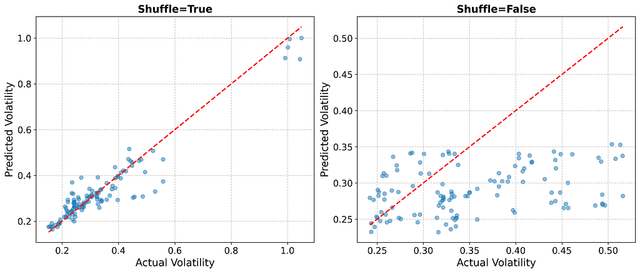